Pharmaceutical Formulation Manufacturing Company
Module: Production Planning and SchedulingIndustry: PharmaceuticalsSchedule a FREE Demo
Client Overview: Large Pharma focused on APIs and Formulation and Injectables primarily focused on the exports market.Client has 13 factories, 10 (including 2 API plants) in India and the rest located in UK / Mexico. It has over 10,000 product registrations worldwide with leadership in key therapeutic segments like CNS, Cardiovascular, Diabetology, Gastroenterology, Urology and Oncology.
Key Features
- Simultaneous Capacity / Material Planning
- Priority, Batch Sizes, Lead Time, Shelf Life
- Sequence Dependency
- Optimization for MRP Supplier / BOM Selection
- Layout Optimization of Packing Lines

Problem Method and Result
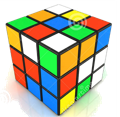
The Puzzle
- The client has a large number of manufacturing facilities. They wanted to focus on two of their large formulation plants.
- The requirement was to plan the production across the process from Granulation – Coating – to HALB and from there to Packing.
- In addition, the client also wanted the MRP to incorporate key additional logic for Batch Selection and Supplier selection.
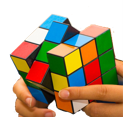
The Solution
- Production Planning and Scheduling was implemented take care of the entire manufacturing process from Granulation / Coating to Packing.
- On the Material constraints, we implemented batch sizes, supplier lead times, shelf life raw material logic.
- SFG / HALB level planning was done with HALB considered as a decoupling point.
- On the Capacity constraints, campaign management and sequence dependent set up times ensured lower change over times, HALB level scheduling, packing line optimization.
- Advanced MRP was implemented incorporating BOM selection and Supplier Selection logic.
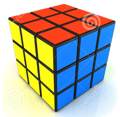
The Result
- Better visibility of the Production.
- Improved Productivity and Capacity Utilization.
- Greater Inventory Control.
- With MRP+, optimal BOM and Supplier Selection was attained to achieve lower inventories at RM / WIP / FG stage.
- Considering HALB as a decoupling point ensured that FG demand variabilities were cushioned using HALB inventory.
- Packing Lines were optimized to reduce Packing Line changeovers.

More Case Studies
Crompton
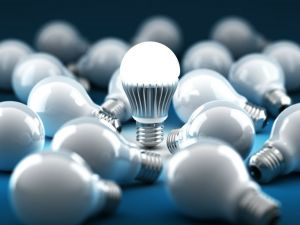
ATG
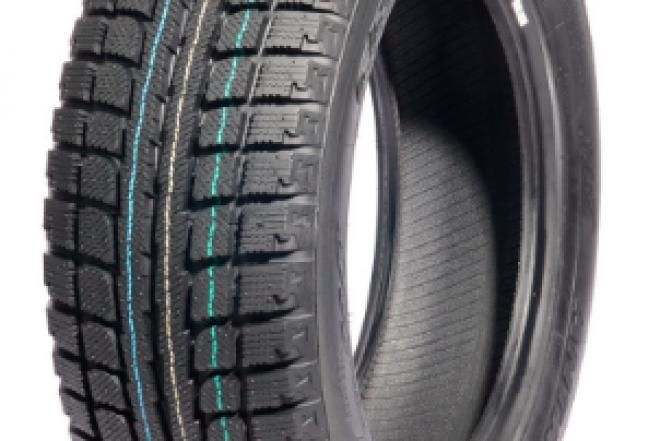

Related Products
S Suite
A Suite
V Suite
I Suite

Related Products
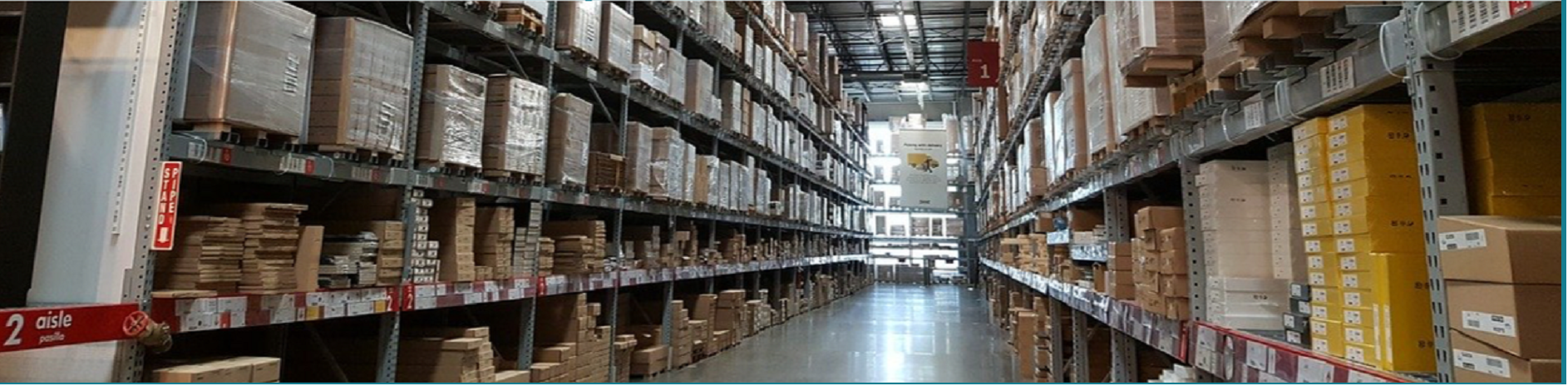
Replenishment Planning
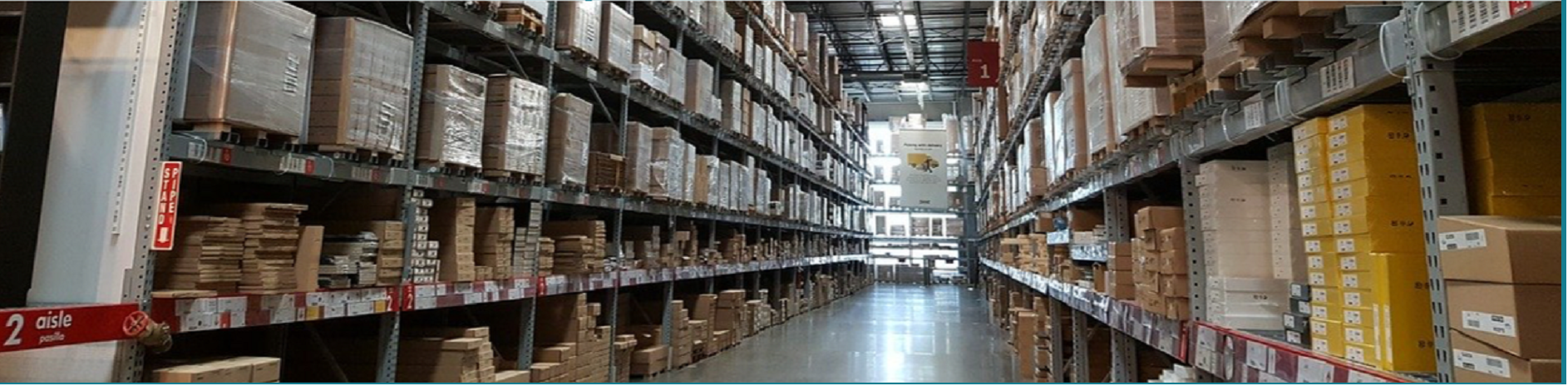
Demand Forecasting
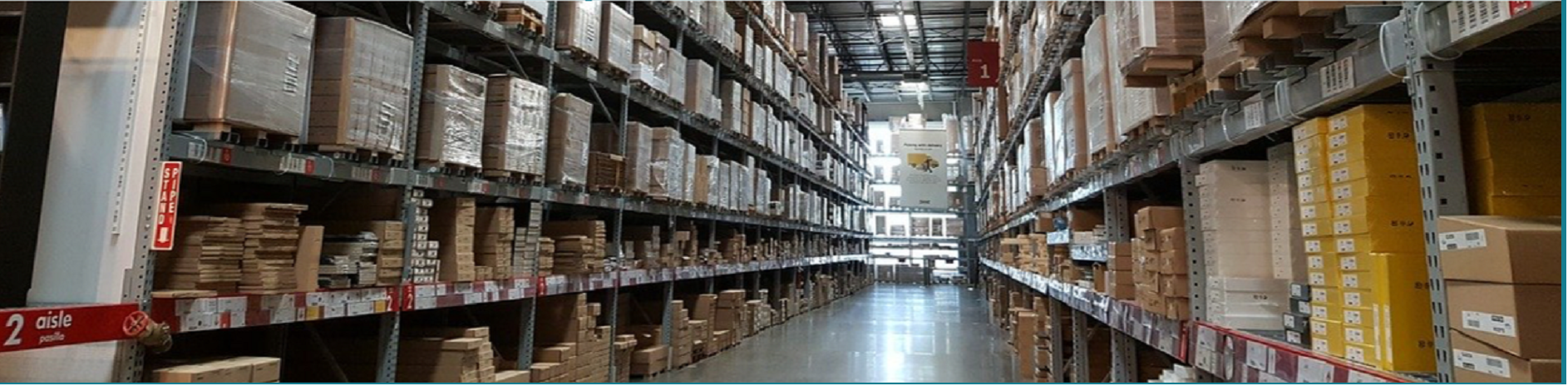
Dashboards
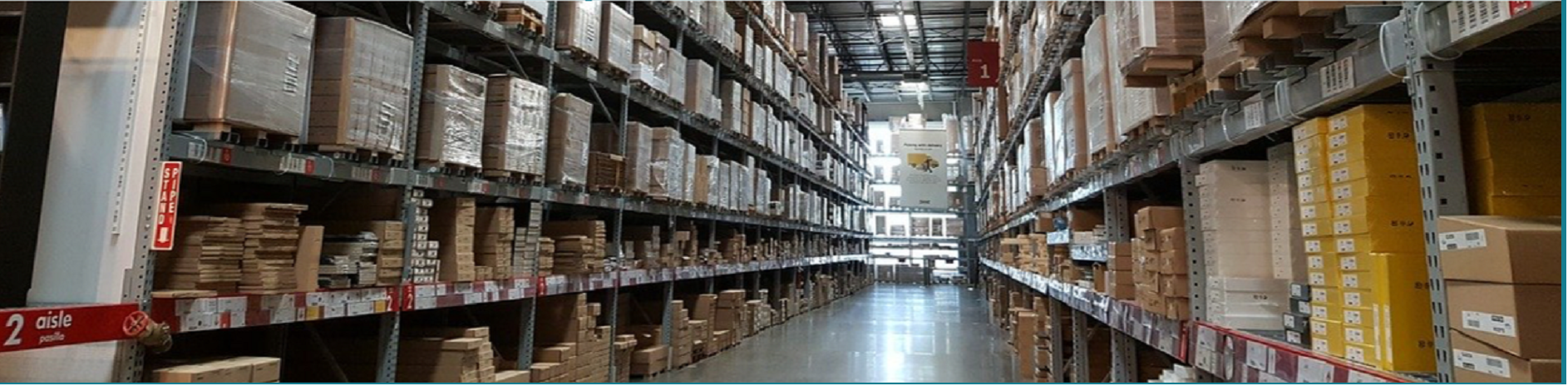
Production Planning and Scheduling

Address
3rd Floor Stellar tower, Sion Panvel Road,Opposite K Star Mall, Chembur, Mumbai,Maharashtra 400071Contact
info@inquizity.com+91-9833257112Copyright
Copyright (c) 2020 Inquizity Metanoia OPC Pvt. Ltd.GST: 27AAECI6558A1ZNSchedule a FREE Demo
Fabric & Domestic Cleaning Products Company
SOLUTIONS DESIGNED TO SYSTEMATICALLY ESTIMATE MARKET DEMAND
Be it New Product Development, Planning, or Promotions, implementing collaborative Demand Forecasting provides you with statistical / ML / AI data to support the course of action in the medium term.
the puzzle
Demand Planning comprises key step of arriving at a baseline number. This is done by using Statistical and ML/AI methods. The Module applies various methods of forecasting on the historical Sales data to arrive at the forecast in the coming months. The forecasting can be done at various levels such as SKU-Depot / Brand-Region / SKU Region
the solution
the result
Multiple Heirarchy
Forecasting at multiple Hierarchies e.g. SKU-Location, SKU-Sales Office, Brand-Channel etc.
Supersession
Supersession that allows linking of old to new items to provide meaningful continuity in history for effective forecasting new product
Statistical Methods
Variety of Forecasting Techniques using efficient Python to take care of different distributions such as seasonality, promotions etc. Also allows for Outlier Detection
Classification of Products
Dynamic Classification of Products across Runner / Repeater / Stranger using different parameters of frequency, variability etc.
ML / AI Methods
Selection of different AI / ML Methods for cases where ML can be implemented
Choice of Error Methods
Selection of Techniques based on different Error Measures with ability to add custom Statistical Techniques